Overview
Welcome to the 2nd Workshop on AI for Content Generation, Quality Enhancement and Streaming at ICCV 2025 (Hawaii) 🌴🌅🌊
After the success of the 1st edition at CVPR 2024... we repeat and improve location!
This workshop focuses on unifying new streaming technologies, computer graphics, and computer vision, from the modern deep learning point of view. Streaming is a huge industry where hundreds of millions of users demand everyday high-quality content on different platforms.
Computer vision and deep learning have emerged as revolutionary forces for rendering content, image and video compression, enhancement, and quality assessment.
From neural codecs for efficient compression to deep learning-based video enhancement and quality assessment, these advanced techniques are setting new standards for streaming quality and efficiency.
Moreover, novel neural representations also pose new challenges and opportunities in rendering streamable content, and allowing to redefine computer graphics pipelines and visual content.
2024 Photo Gallery & Slides (CVPR)
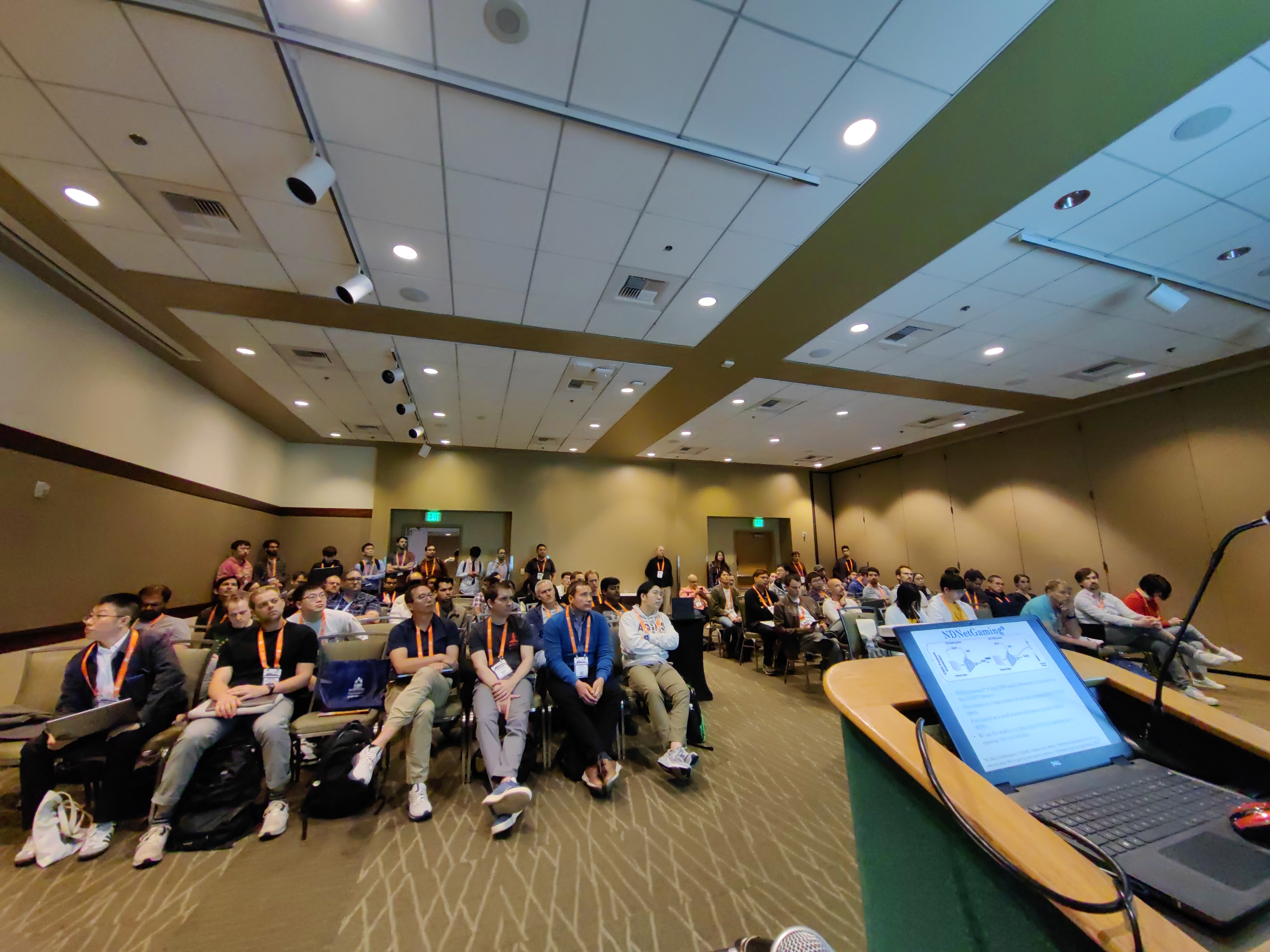
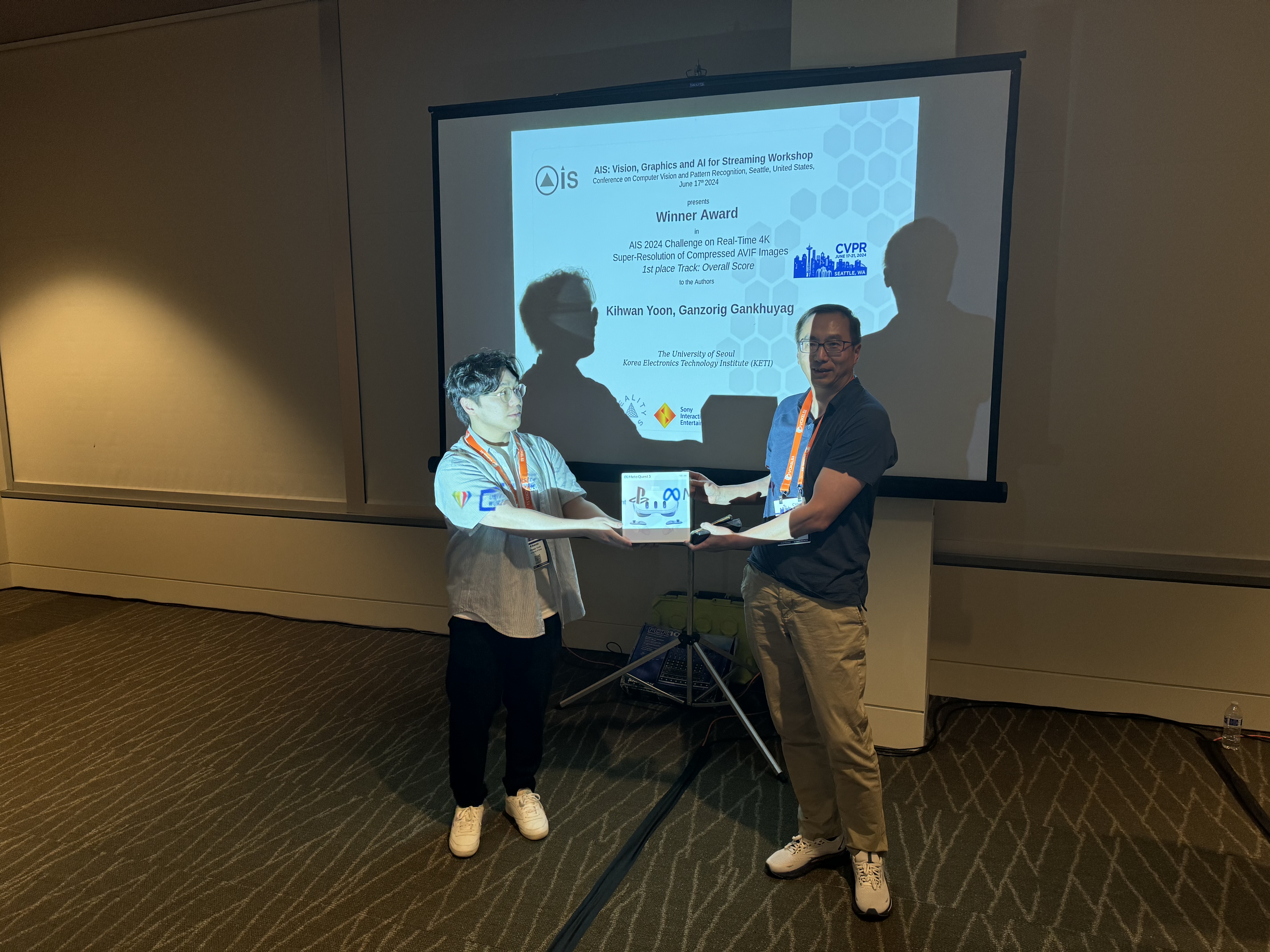
Call for Papers
We welcome papers addressing topics related to VR, streaming, efficient image/video processing and compression. The topics include:- Efficient Computer Vision
- Model optimization and Quantization
- Image/video quality assessment
- Image/video super-resolution and enhancement
- Compressed Input Enhancement
- on-device & edge processing
- Generative Models (Image & Video)
- DeepFakes
- Vision Language Models
- Real-time Rendering
- Neural Compression
- Computer Graphics
Submission site: https://cmt3.research.microsoft.com/AIGENS2025
Submission Guidelines
- A paper submission has to be in English, in pdf format, and at most 8 pages (excluding references) following ICCV style. The paper format must follow the same guidelines as for all ICCV 2025 submissions https://iccv.thecvf.com/Conferences/2025/AuthorGuidelines
- Dual submission is not allowed. If a paper is submitted also to ICCV and accepted, the paper cannot be published both at the ICCV and the workshop. If the paper is under review elsewhere, it cannot be submitted here.
- The review process is double blind. Authors do not know the names of the chair/reviewers of their papers. Reviewers do not know the names of the authors.
Important Dates (TBU)
Regular Paper submission deadline (& ICCV resubmissions)    | 1st July, 2025 |
Challenge Paper submission deadline (only challenge solutions)    | 8th July, 2025 |
Paper decision notification (firm)    | 11th July, 2025 |
Camera ready deadline (firm)    | 18th August, 2025 |
CMT Acknowledgement (click)
The Microsoft CMT service was used for managing the peer-reviewing process for this conference. This service was provided for free by Microsoft and they bore all expenses, including costs for Azure cloud services as well as for software development and support.Challenges 🚀
We will host multiple challenges focused on realistic image/video applications
Challenges are in the making, will end middle/end July
The top ranked participants will be awarded and invited to present their solution at the AIGENS workshop at ICCV 2025.
The challenge reports (if applicable) will be published in the ICCV 2025 conference proceedings.
The participants can also submit papers describing their solution to the challenges and/or related problems.
Invited Speakers
Schedule Details - TBD, Full-Day, 20th October
Organizers
Program Committee
Radu Timofte (University of Würzburg)
Eduard Zamfir (University of Würzburg)
Jingzhi Li (University of Würzburg)
Jonas Brenig (University of Würzburg)
Tim Seizinger (University of Würzburg)
Ioannis Katsavounidis (Meta)
Ryan Lei (Meta)
Cosmin Stejerean (Meta)
Zhi Li (Netflix)
Ren Yang (Microsoft)
Saman Zadtootaghaj (Sony Interactive Entertainment)
Nabajeet Barman (Sony Interactive Entertainment)
Julian Tanke (Sony AI)
Takashi Shibuya (Sony AI)
Yuki Mitsufuji (Sony AI)
Past Invited Speakers
Past/Present Sponsors, Organizers and Collaborators
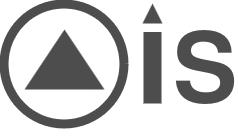
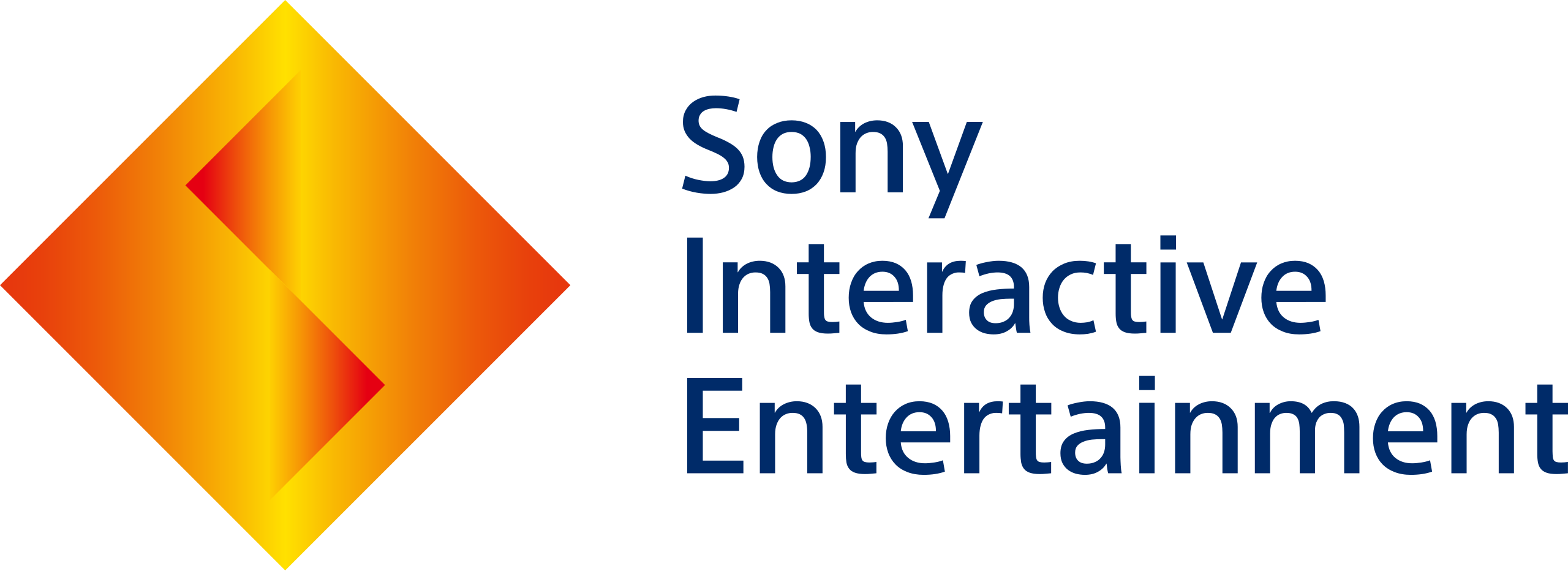
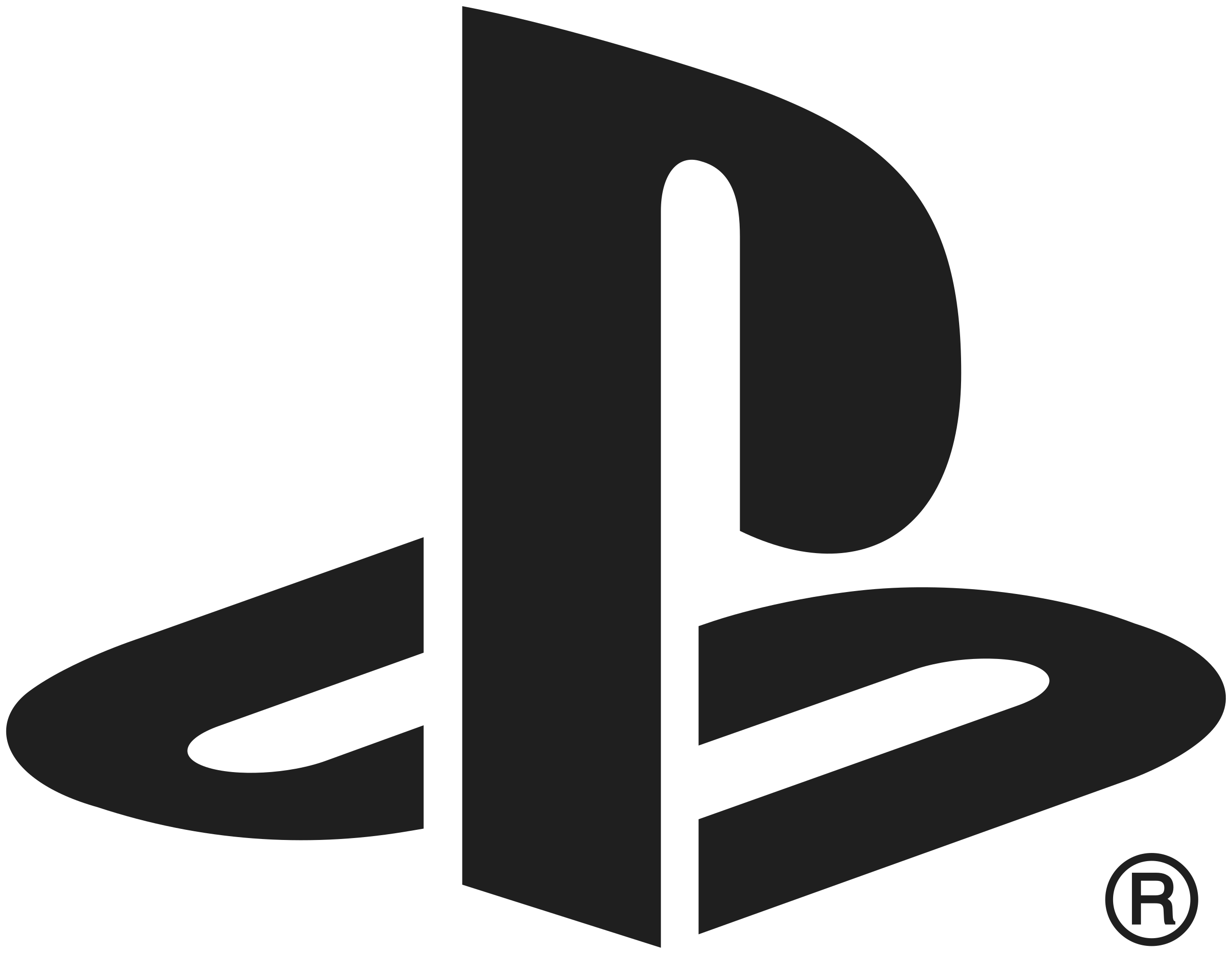
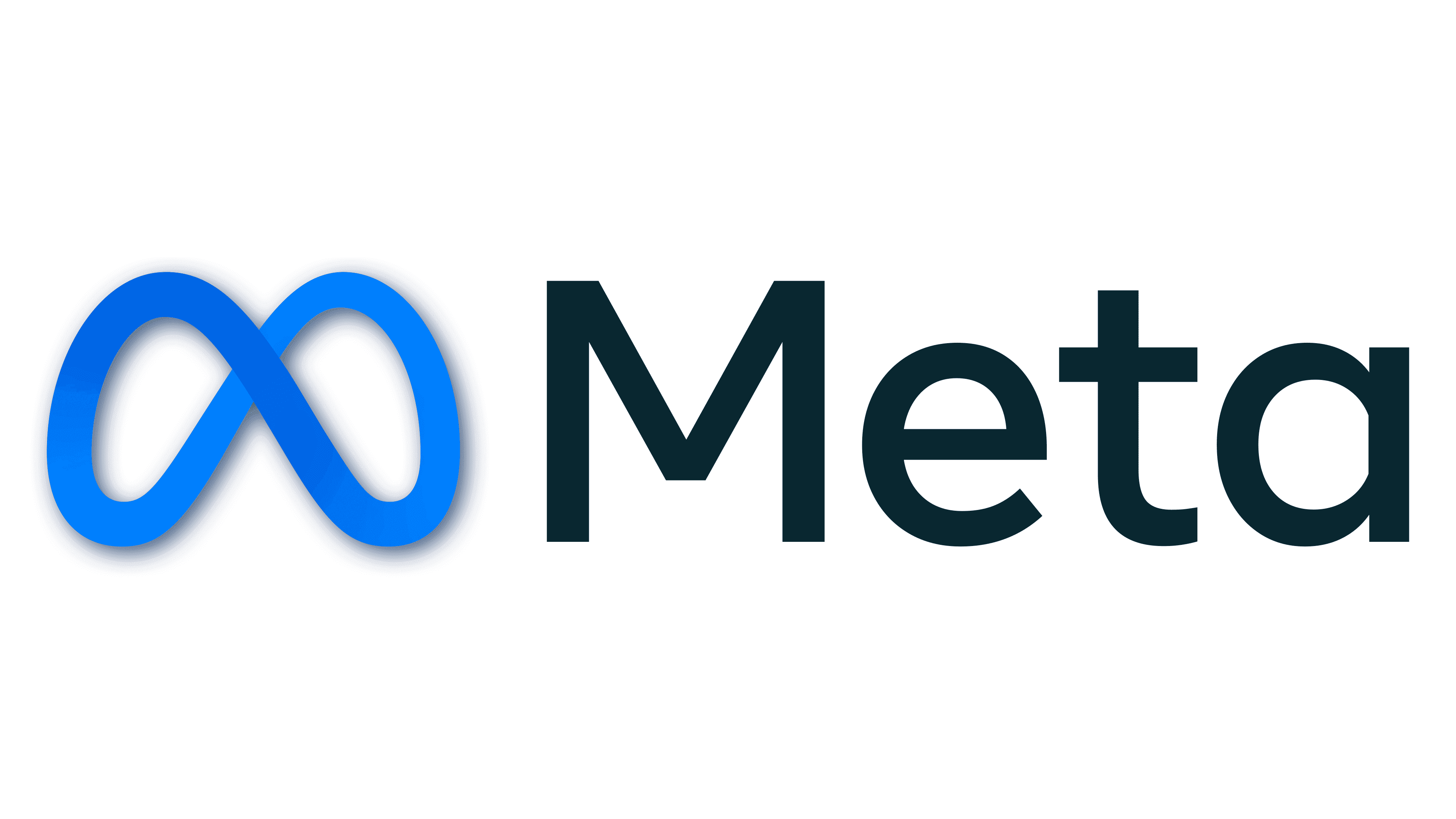
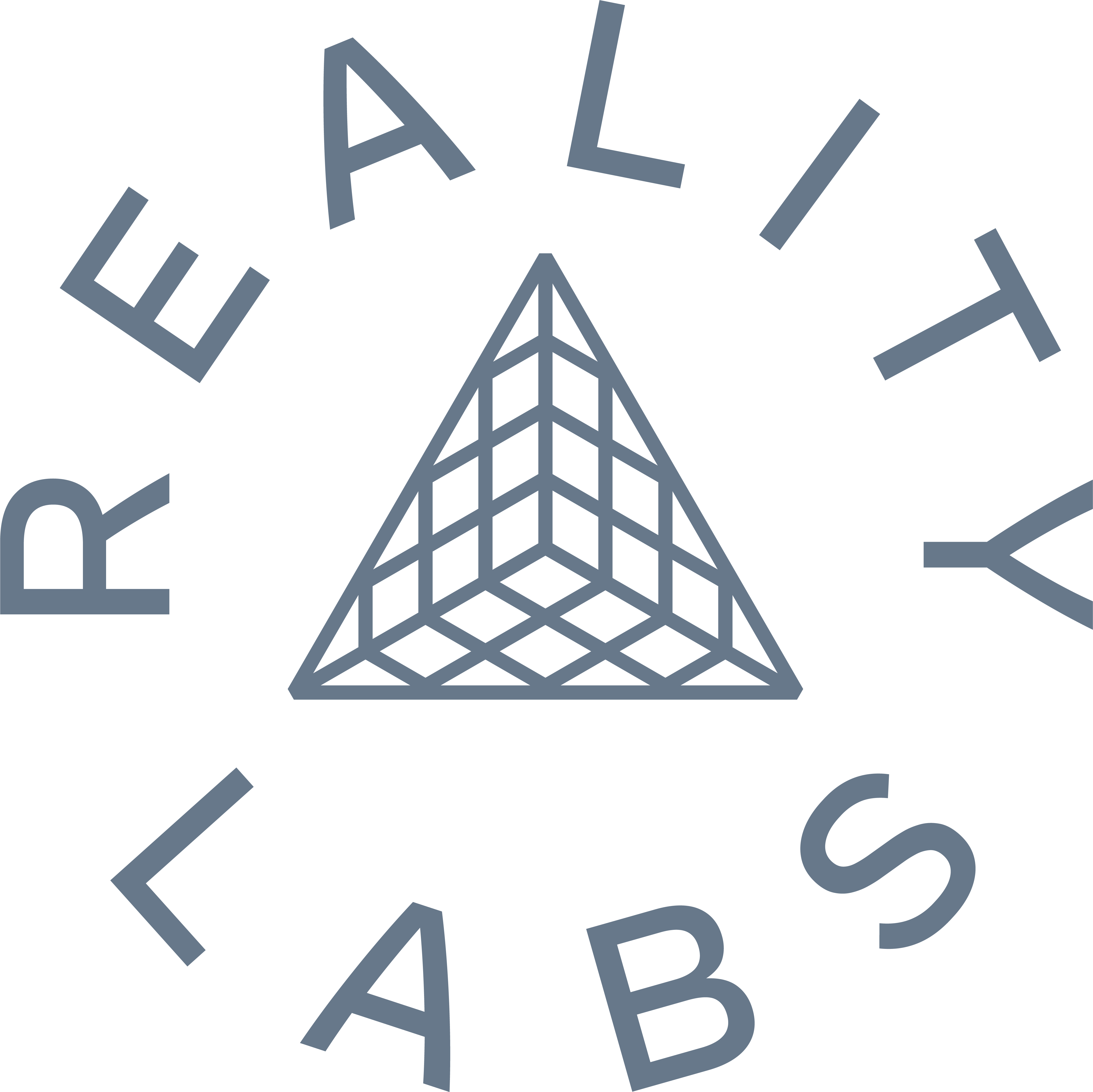
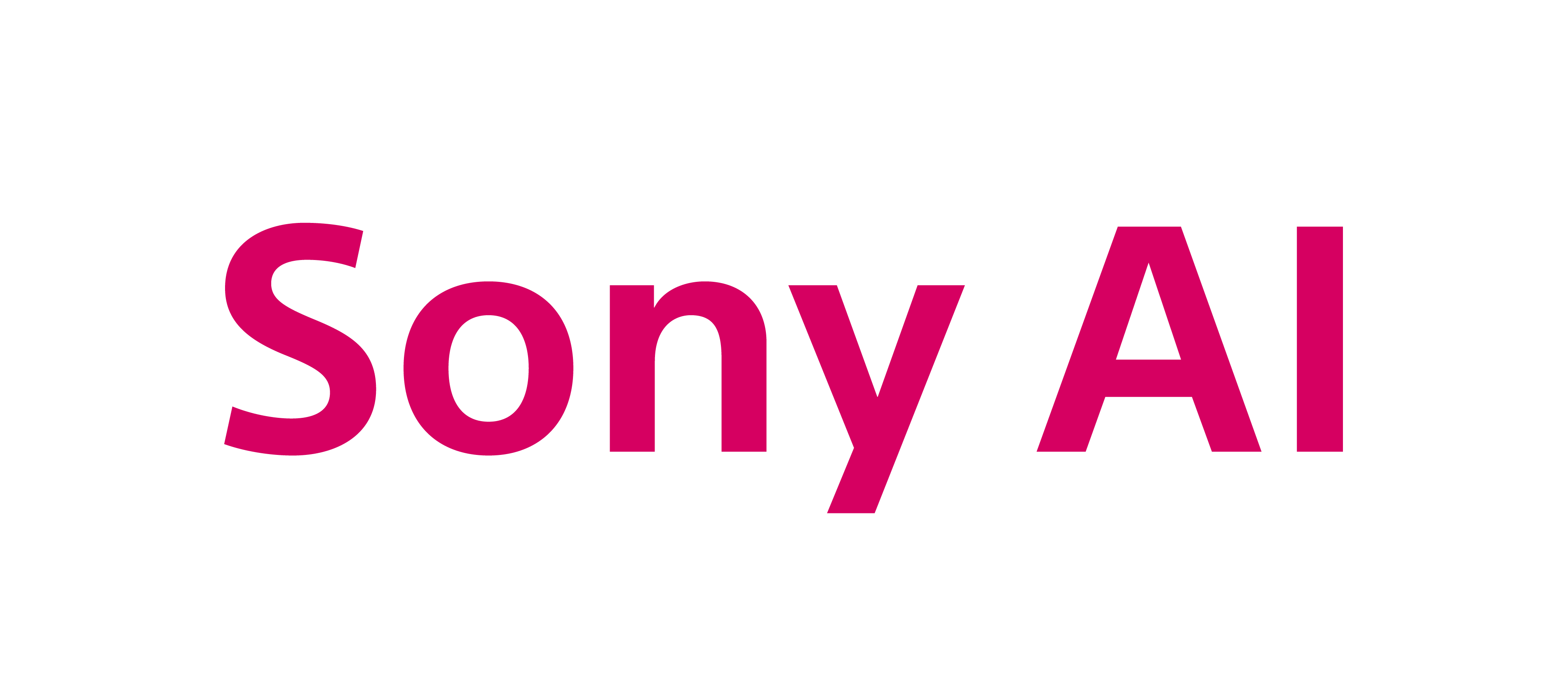
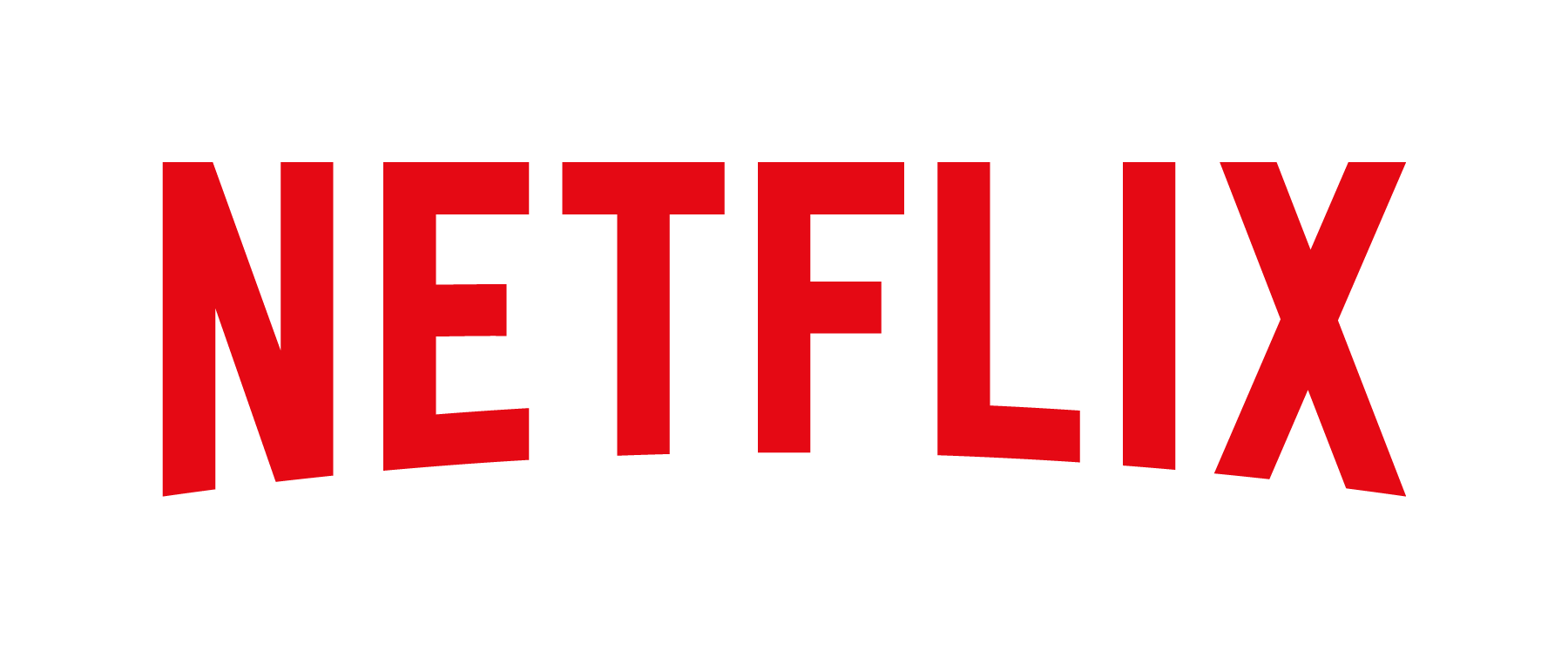
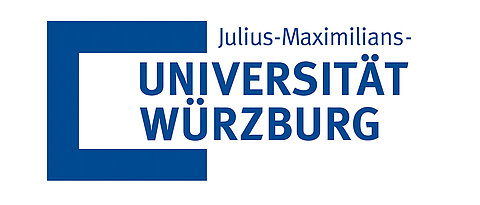
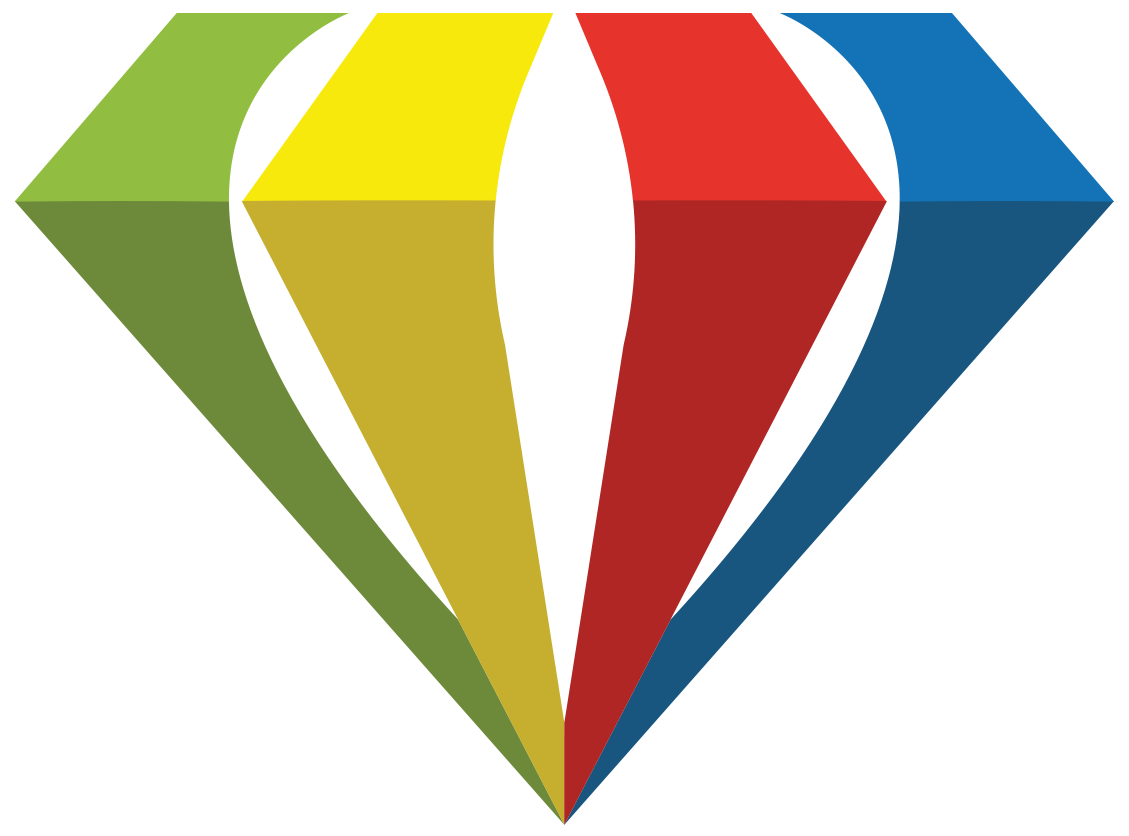